“A truly wise person is not someone who knows everything, but someone who is able to make sense of things by drawing from an extended resource of interpretation schemes.”
Sönke Ahrens, How to Take Smart Notes (2017, pg. 116)
Everything we know about the world is a model, a simplified representation of something. This includes every word, language, map, math equation, statistic, document, book, database, and computer program, and our “mental models.”1 We use models constantly to simplify the world around us, to create knowledge, and to communicate. Our mental models, the collection of theories and explanations that we hold in our heads, provide the foundation upon which we interpret new information.
The process by which we create models is called “abstraction,” which helps us approximate complex problems with solvable ones that are simple enough to (hopefully) enable us to make a decision or find an answer. Our human ability to create useful abstractions (knowledge) is the reason why we are such a successful species on Earth.
However, we seldom think about how we fail at abstraction, or how to do it better. We commit abstraction errors whenever we over-simplify or over-complicate problems. We fail by trying to remember isolated facts, instead of trying to understand concepts and draw meaningful connections with other ideas. Finally, we place undue faith in our preferred models by failing to recognize that all models are—as we shall see—wrong.
Practicing good abstraction can help us generate truly creative solutions, while avoiding costly mistakes.
“Consider a spherical cow”
“Any model… must be an over-simplification. It must omit much, and it must over-emphasize much.”
Karl Popper, The Myth of the Framework (1994, pg. 172)
In order to be useful, models must ignore (abstract away) certain variables or features, in order to highlight others.
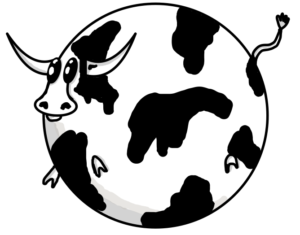
An old joke from theoretical physics involves a dairy farmer struggling with low milk production. Desperate, the farmer asks for help from the local academics. A team of theoretical physicists investigates the farm and collects vast sums of data. Eventually, the leader returns and tells the farmer, “I have the solution, but it works only in the case of spherical cows in a vacuum.”
The metaphor refers to the tendency of physicists (and other scientists) to use models and abstractions to reduce a problem to the simplest possible form to enable potentially helpful calculations, even if the simplification abstracts away some of the model’s similarity to reality.2
Abstract thinking requires a balancing act: we must be rigorous and systematic, but also make conscious tradeoffs with realism. We isolate a simple part of the problem, abstracting away all irrelevant details, and calculate the answer. Then, we put our “spherical cow” answer back into context and consider whether other factors might be important enough to overturn our conclusions.
Zooming in and out
Imagine how impossible it would be if we could only learn reductively—that is, by analyzing things into their constituent parts, such as atoms. The most basic events would be overwhelmingly complex. For example, if we put a pot of water over a hot stove, all the world’s supercomputers working for millions of years could not accurately compute what each individual water molecule will do.
But reduction is not the only way to solve problems. Many incredibly complex behaviors cannot be simply “derived” from studying the individual components. We can’t analyze one water molecule and predict the whole pot’s behavior. Good explanations, it turns out, can exist at every abstraction layer, from individual particles to whole systems and beyond! High-level simplicity can “emerge” from low-level complexity.3
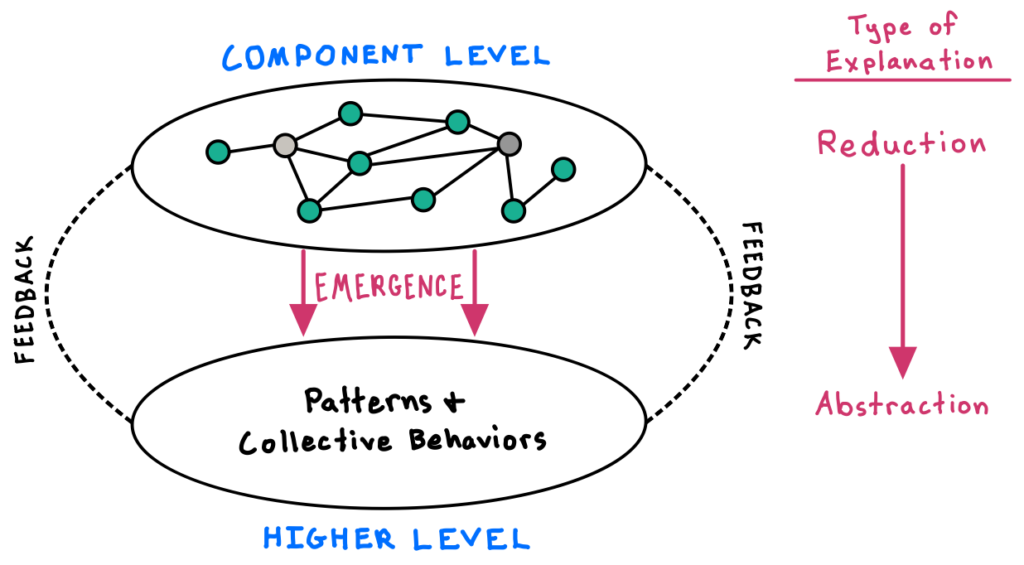
Let’s revisit our boiling water pot. Predicting the exact trajectory of each molecule is an impractical goal. If we were instead concerned with the more useful goal of predicting when the water would boil, we can turn to the laws of thermodynamics, which govern the behavior of heat and energy. Thermodynamics can explain why water turns into ice at cold temperatures and gas at high ones, using an idealized abstraction of the pot that ignores most of the details. When the water temperature crosses certain critical levels, it will experience phase transitions of freezing, melting, or vaporizing.4 The laws of thermodynamics themselves are not reductive, but abstract; they explain the collective behaviors of lower-level components such as water molecules.
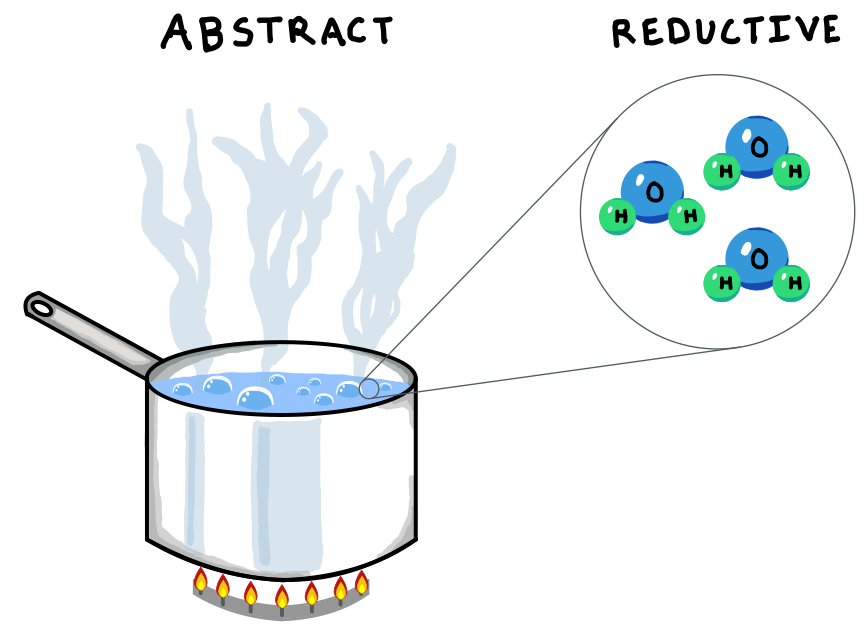
We can use this trick of “zooming” in and out between abstraction levels to generate creative explanations and solutions in many contexts, including in business. For instance, if we only analyzed our business at the company-level, we risk ignoring key factors that emerge only at higher levels (such as the industry or the economy) or at lower levels (such as a product, team, individual, or project).
There is no “correct” level of analysis. A good strategist considers various perspectives in order to understand the true nature of the problem and triangulate on the highest-leverage places for intervention.
Wrong models and doomsday prophecies
“All models are wrong, some are useful.”
George Box, statistician
By simplifying things, models help us reach a decision even when we have limited information. But because they are simplifications, models will never be perfect. Consider the following examples:
- Résumés and interviews are (flawed) models to predict a candidate’s success in a role.
- Maps helps us navigate the world by simplifying its geography. (Imagine how useless a “perfect,” life-sized map would be.)
- Statistical models such as the normal distribution and Bayesian inference can help us make data-driven decisions, but they require us to make a bunch of iffy assumptions and simplifications.
- Our best economic models are potentially accurate in some contexts, and wildly inadequate in others.
Failing to recognize the limitations of our models can lead to massive errors.
In 1798, the famous population theorist Thomas Malthus created a model of population growth and agricultural growth which led him to prophesy that the 19th century would bring about mass famine and drought. The model asserted that because the population grows exponentially while sustenance only grows linearly, humanity would soon outgrow its ability to feed itself. Malthus and many others thought he had discovered an inevitable end to human progress.
In hindsight, Malthus’s population projections were pretty accurate, but due to a critical error of abstraction, he wildly underestimated agricultural growth, which actually outpaced population growth. His simplified model abstracted away a variable that turned out to be pretty important: humans’ ability to create new technology.5 Indeed, we achieved incredible agricultural advances during this time, including in plows, tractors, combine harvesters, fertilizers, herbicides, pesticides, and improved irrigation.6 Today, famine mortality rates are a fraction of what they were when Malthus made his pessimistic prophesy, even though the global population is 10x larger.
Not only are models inherently imperfect, but also we can never be 100% certain that our model is the best one to use in the first place!7 We must not let our current models shut out fresh ideas or alternative explanations. The ongoing replacement of our best theories and models with new and better ones is not a bug of the knowledge creation process, it is a feature.8
For instance, Einstein’s groundbreaking theory of general relativity ended the 200-year reign of Isaac Newton’s theory of gravity. Already, we know that general relativity and quantum theory, our other deepest theory in physics, are completely incompatible with one another. One day, both will be replaced.
***
Once we accept that models are valuable yet imperfect and impermanent, we can harness them to our advantage. Good strategists can generate creative solutions by analyzing complex problems from various perspectives, perhaps reframing the issues altogether, and by carefully considering which factors are critical and which ones can be safely abstracted away. And we can use our models the right way: as potentially helpful tools in certain circumstances, not as ultimate truth.
References
- Meadows, D. (2008). Thinking in Systems: A Primer. Chelsea Green Publishing. 86-87.
- Krauss, L. (2007). Fear of Physics. Basic Books. 3-8.
- Deutsch, D. (2011). The Beginning of Infinity. Penguin Books. 107-112.
- Bahcall, S. (2019). Loonshots. St. Martin’s Press. 153-159.
- Deutsch, D. (2011). 205-206.
- Bellis, M. (February 6, 2021). American Farm Machinery and Technology Changes from 1776–1990. ThoughtCo.
- Kay, J. (2010). Obliquity. The Penguin Press. 116-120.
- Popper, K. (1963). Conjectures and Refutations. Routledge & Kegan Paul. 124-129.