A local optimum is a solution that is optimal within a neighboring set of candidate solutions—a point from which no small change can generate improvement. However, this local peak may still be far from the global optimum—the optimal solution among all possible solutions, not just among nearby alternatives.
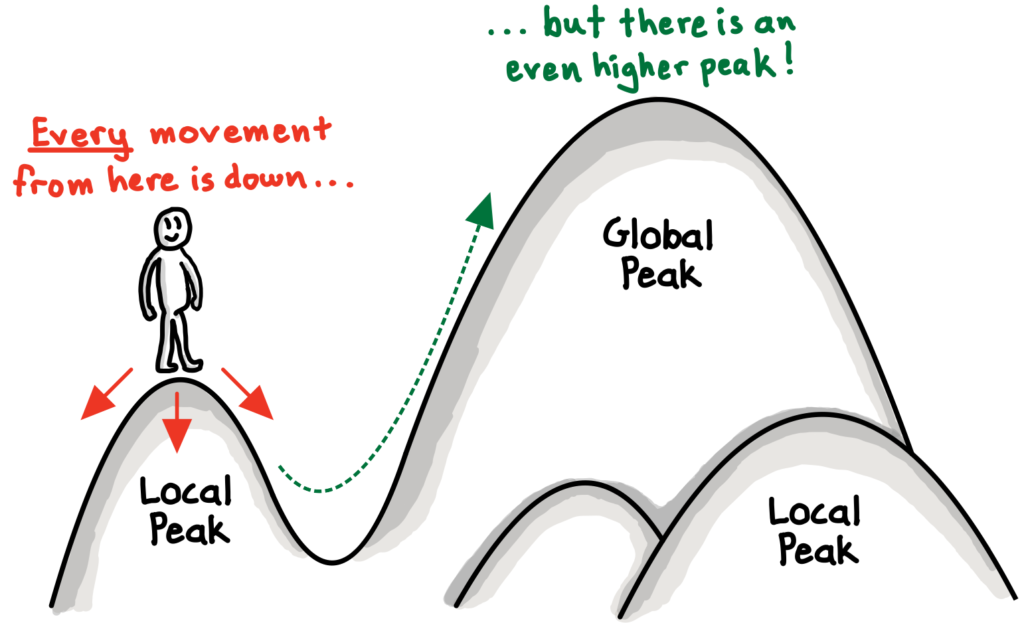
This valuable model can teach us about the inherent tradeoff between capitalizing on our current opportunities and pursuing new ones—whether in biological ecosystems, businesses, or machine learning. We can use it to better understand the complex environments we operate in, and to design more effective strategies to achieve our goals.
Getting stuck
Picture a rugged plane comprised of many peaks and valleys of various elevations, with numerous individuals or groups competing to reach the highest peaks. Nearby points tend to have similar levels of “fitness.” The landscape itself may shift dynamically, altering the peaks and valleys and transforming the available paths to reach them. This model is known as a “fitness landscape,” an extremely useful metaphor for thinking about optimization amidst local and global peaks in a variety of applications—including systems, biology, computer science, and business.1
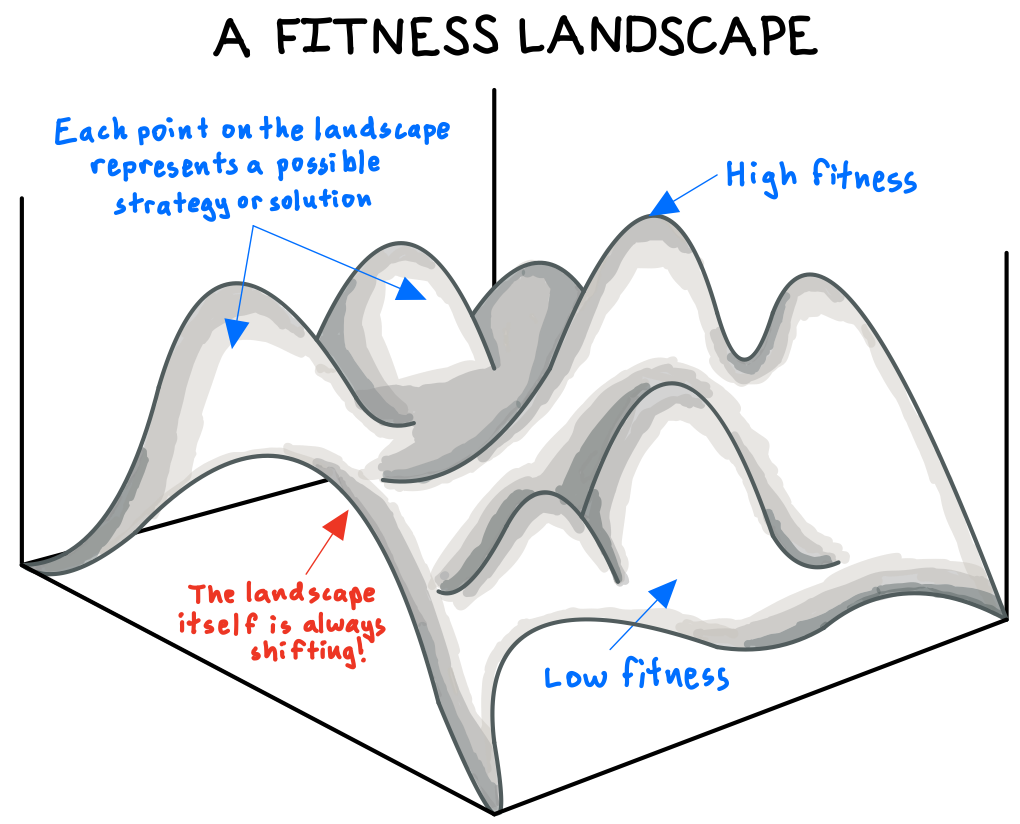
In complex systems (such as an industry or an ecosystem), it is easy to get stuck on local peaks as the ground shifts beneath our feet (undermining our position), especially if we fail to survey new territory. We won’t know precisely how the landscape will shift, so the only way to sustain progress in the long-term is, simply, to explore.
Sometimes, we may even have to go down (temporarily worsen our situation) in order to ascend a higher peak. And this requires a lot of courage. For example, Netflix’s stock fell by almost 80% from its peak after CEO Reed Hastings announced they were getting out of the DVD business in 2011. Ten years later, Netflix had pioneered the video streaming industry, and its stock price had grown by nearly 1,300%!
Evolutionary searches can never relax. We must constantly experiment with new ideas and strategies to find better solutions and adapt as the landscape shifts.
Faster than the speed of evolution
In biological evolution, we can visualize the competition for genetic dominance as a rugged fitness landscape in which the peaks and valleys represent the highs and lows of evolutionary fitness across an ecosystem. Higher peaks represent species or organisms that are better adapted to their environment—that is, ones that are more successful than their nearby competitors at causing their own replication.
Evolution is capable of creating remarkably complex and useful features, such as the human body’s ability to repair itself or the peacock’s brilliant tail. However, because it optimizes only for the ability of genes to spread through the population, evolution will inevitably reach only local peaks of fitness within a given environment.2 It can favor genes that are useless (the human appendix), suboptimal (women’s narrow birth canals), or even destructive to the species. For instance, the peacock’s large, colorful tail that helps it find mates also makes it more vulnerable to predators.3
When the landscape shifts, even a highly adapted species will be unable to evolve toward a worse (less well-fitted) state than its current one in order to begin ascending a new, higher evolutionary peak. If the environment shifts faster than the species can adapt to it, mass extinctions can occur.4
Fortunately, we humans don’t need to be bound by evolutionary timescales. Often, we can find better hills to climb.
Let’s look to computer science and business to see why.
Getting un-stuck
Algorithms provide useful insights into optimization and into overcoming local peaks.
The simplest optimization algorithm is known as “gradient ascent,” in which the program just keeps going “up.” For instance, a video site such as YouTube might be programmed to continue recommending videos that resemble your past content consumption. But “dumb” algorithms like this one maximize only short-term advantage, leading us to local peaks but not to global ones. What if the user’s content preferences change? What if the viewer gets bored by stale recommendations? What if repetitive videos trap the user in a filter bubble?
Randomness and experimentation can help us “pogo-jump” to higher peaks that simple gradient ascent would not reach. For example, a “jitter” involves making a few random small changes (even if they seem counterproductive) when it looks like you are stuck on a local peak, then resuming hill-climbing. A “random-restart” involves completely scrambling our solution when we reach a local peak—which is particularly useful when there are lots of local peaks.5
Perhaps our video site should recommend random pieces of viral content even if the viewer hasn’t watched similar clips previously. Or show clips that contrast sharply with past viewing habits (for nuance or contrarian content). Only experimentation can reveal whether we are climbing the best hill.
The explore/exploit tradeoff
In business, it is useful to picture the strategic environment as a rugged landscape, with each “local peak” representing a coherent bundle of mutually reinforcing choices.
Every organization needs to balance experiments in exploitation of its current businesses with experiments in exploration for future innovations. In the short-term, simple “gradient ascent” strategies (keep going up) help ensure the company is exploiting its current strengths and opportunities. Over the long-term, however, companies must make occasional medium- or long-distance “pogo jumps” to prevent getting stuck on local peaks and, sometimes, to make drastic improvements. The key problem with many organizations is that when the environment seems stable, they stop experimenting because it seems costly and inefficient, and because it sometimes creates internal competition.1
This was Reed Hastings’s revelation about Netflix in 2011: its wildly successful DVD-by-mail business was merely a local peak. The landscape had shifted. The new global peak, he believed (correctly), was streaming.
***
The overall lesson is that because the environment is uncertain and always changing, good strategy requires individuals and organizations to carefully cultivate and protect a portfolio of strategic experiments, creating valuable options for the future.
Even when it seems we are at a “peak,” there may be even higher peaks that we cannot yet see, and the peaks themselves are constantly shifting! In such an environment, complacency is a death sentence.
Notes
- Beinhocker, E. (January 1999). On the origin of strategies. The McKinsey Quarterly, 4(4), 169-173.
- Deutsch, D. (2011). The Beginning of Infinity. Penguin Books. 79-81, 106.
- Deutsch, D. (2011). 89-93.
- Gribbin, J. (2004). Deep Simplicity. Random House. 206-207.
- Christian, B., & Griffiths, T. (2016). Algorithms to Live By. Henry Holt and Co. 194-197.